Continuous test data
Provisioning test data in a Continuous Testing world
IT is evolving rapidly. A couple of years ago we only talked about ‘The Cloud’, nowadays we talk about machine learning and AI. With all these new challenges, your applications are undergoing constant transformation or, at the very least, being adequately maintained. Adding new features and/or maintaining current application software remains important because these core systems are the backbone of your organization. If you’re able to address these changes quickly you’re one step ahead of your competitors.
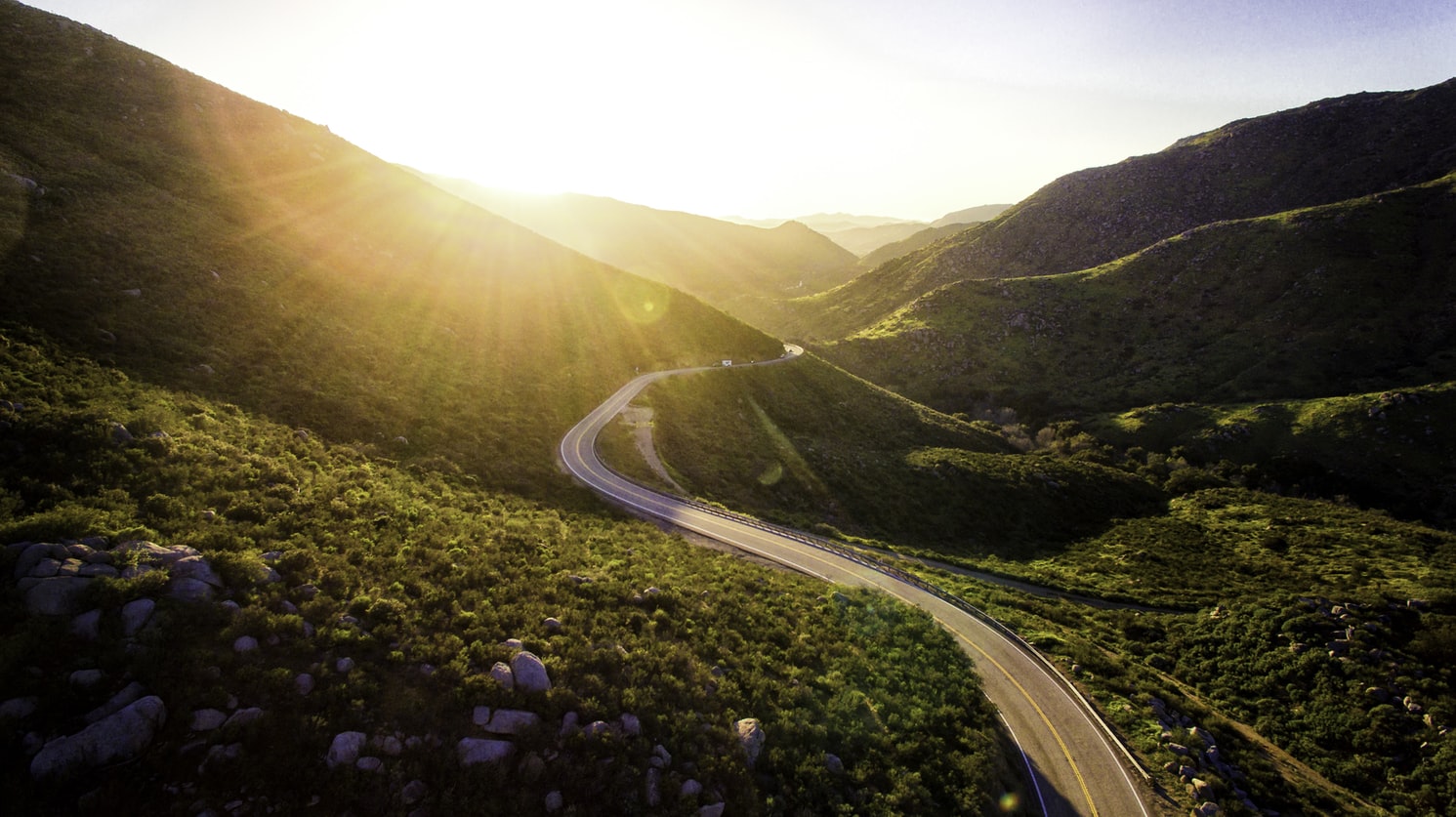
Addressing changes to your applications quickly is important. This is one of the reasons why organizations adopted the agile ideology. One of the important parts in this whole (agile) cycle is the use of many smaller feedback loops in favour of one major feedback loop. To create such a process, organisations are adopting what we now know as CI/CD – Continuous Integration and Continuous Delivery (or Deployment). This means that the testing processes must happen earlier and more frequently in the development cycles and so we call it Continuous Testing..
That’s how Continuous Testing has evolved, but what is Continuous Testing and how does test data play a part in the process?
What is Continuous Testing?
“Continuous Testing is defined as a software testing type that involves a process of testing early, testing often, test everywhere, and automate. It is a strategy of evaluating quality at every step of the Continuous Delivery Process. The goal of Continuous Testing is test early and test often. The process involves stakeholders like Developer, DevOps, QA and Operational system” Source: Guru99.
What do you need for continuous testing?
First of all Continuous Testing is not necessarily the same as test automation. Test automation is a prerequisite for Continuous Testing and is part of something bigger. In the same article the differences are noted:
Definition of Test Automation:
Test automation is a process where a tool or software is used for automating tasks.
Definition of Continuous Testing:
It is a software testing methodology which focuses on achieving continuous quality & improvement.
To achieve Continuous Testing the first thing you’ll need is test automation. According to the same article the way to go is:
1. Using tools to generate test automation suite from user stories/requirements
2. Create a test environment
3. Copy and anonymize production data to create test data set
4. Use service virtualization to test API
5. Parallel performance testing
What’s striking in this list is the statement that you need to copy and anonymize production data to create a test data set. Ask yourself: Is a full-size copy of production needed? It shouldn’t be necessary. If you’ve got one or multiple small updates of your application you want to test it continuously, preferably against a set of test data that is affected by the update(s). So do you really need that much test data? But what is the impact on Continuous Testing if you have an (anonymized) full sized copy of the production data? Let’s start with this last point.
The impact of a full copy
The impact of having a full copy of anonymized production data on Continuous Testing is no different whether tests are automated or performed manually. It’s a discussion about representativeness and speed. The key consideration is how can you anonymize your test data in a credible, usable manner and how quickly can you make it available? Masking a database will take time and therefore adds to the test data deployment cycle. Anyone who says otherwise has never had to do the job before or they’re making light of an important part of the process. Instead of copying it from production to test, you’ll have a process of copying, masking and then deploying it.
With the advent of data protection legislation across the globe, masking a database is now a mandatory aspect of deploying to the lower environments. The time taken to deploy the masked database is the foundation of the decision on the refresh frequency. Some organization may be able to refresh daily, others less frequently.
One consideration of masked database refreshes is that of the consistency of the masked results. You do not want different masked results on different days so you should ensure that your chosen masking processes will use a consistent masking technique. If you don’t do this you will thoroughly confuse the lower environment users who, one day, are looking at “Mary Jones” and on the following day she becomes “Jane Doe”.
Once the first masked database is deployed it can act as a source for subsequent copies within the lower environments. It effectively becomes the “Test Data Master” of scale – full production size.
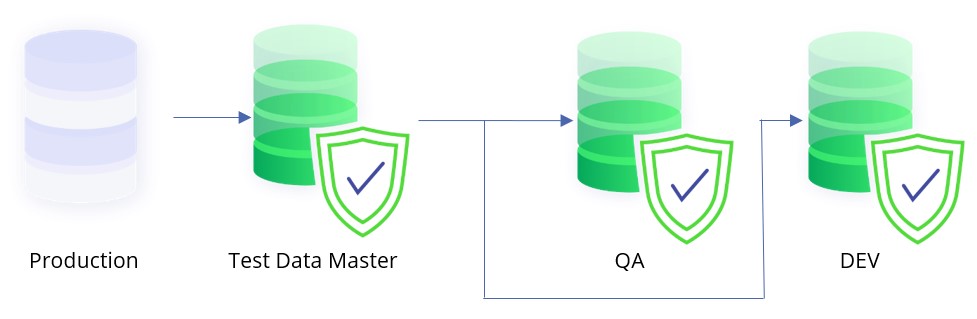
But why make multiple copies of the full scale database? Some would argue that full size is needed for volume testing, typically testing queries with a view to optimizing them – they’re usually the BI team. Others would say that the full database isn’t required because the current sprint is focused upon a particular aspect of the application or has a limited data content requirement.
Making additional full scale copies has a cost. First, it’s the time taken to copy and restore, second it’s the size and cost of the space required for each copy. On-prem databases are often closely controlled since it takes time to acquire and add disk resource. In the Cloud it’s magically there and the only person that knows is the person paying the bill at the end of the month. A person who may well not like surprises…
This is the core of the Continuous Testing discussion about using subsets of the database or database virtualization.
Also read: Test Data Management
Test data in Continuous Testing
Using a full-sized copy with anonymized production data, or only synthetic test data, may not fit the deployment timelines necessary for a Continuous Testing methodology. You’ll need something which results in having representative test data that’s easily available and repeatable.
Another impact of a large database is that it will take quite some time before you discover and find the right test data for your test case and you’ll probably not get it right the first time. As an alternative you could choose to generate specific test cases, but then you have the representativeness discussion. All databases have their anomalies and generating test cases tends to instrument these anomalies out of the test case. You’re effectively cleaning the data from the outset.
Typically each sprint will have its own focus and the inception of a sprint will also define the test data requirement. The answer to the question “What do we need?” is the foundation for the subset data selection criteria for that sprint. Given that each sprint will likely have different focuses, it follows that the subsets can and will be different. The benefit is that each sprint can be serviced uniquely and their test data selection is entirely appropriate for their needs.
The impact of a subset
Smaller databases reduce testing time by their very nature. Smaller databases lend themselves to fast refreshes. Smaller databases are easily adapted to evolving test data requirements.
Creating smaller databases from the consistently masked Test Data Master means you’re able to execute the same test with exactly the same test data repetitively or even incrementally. The added benefit of the full scale Test Data Master is that it can be used by those teams which need the volume experience without impacting others.
This approach lends itself to the immediate feedback loops inherent in the CI/CD stream. Test early and test often, by its nature, implies the need to periodically recover to a known start point. Both subsetting and virtualization achieve this objective.
Another key benefit of using subsets over virtualization is that the Test Data Master and the subsets are distinct entities. One can exist without the other. This is not the case with database virtualization since the virtualized images (effectively diff files) are directly and completely dependent upon the master image being available. They simply cannot exist without it. Should the master image become unavailable every user, or team, connected to it will experience a service interruption. It goes without saying that this has a direct cost to the business.
Separating the Test Data Master and subsets also means that refreshes from Production can be done in a more timely manner since the teams can work on the subsets while the master is in refresh mode. While that’s happening the BI teams can always use a subset, too.
Conclusion
Once established, Continuous Testing improves and accelerates the pace of your CI/CD pipeline. It means that Dev, Test and QA engineers can focus on executing the right tests on the right data in a timely manner.
Creating masked Test Data Masters and their associated subsets can be facilitated and automated using DATPROF’s Test Data Management suite.
Test Data Automation
Getting hold of test data for your project can sometimes be a struggle, a challenge, maybe we should really call it what it is – a problem. So how do we overcome this problem? This is where Test Data Automation will help!